Generative AI Set To Drive Monumental Tech Shifts
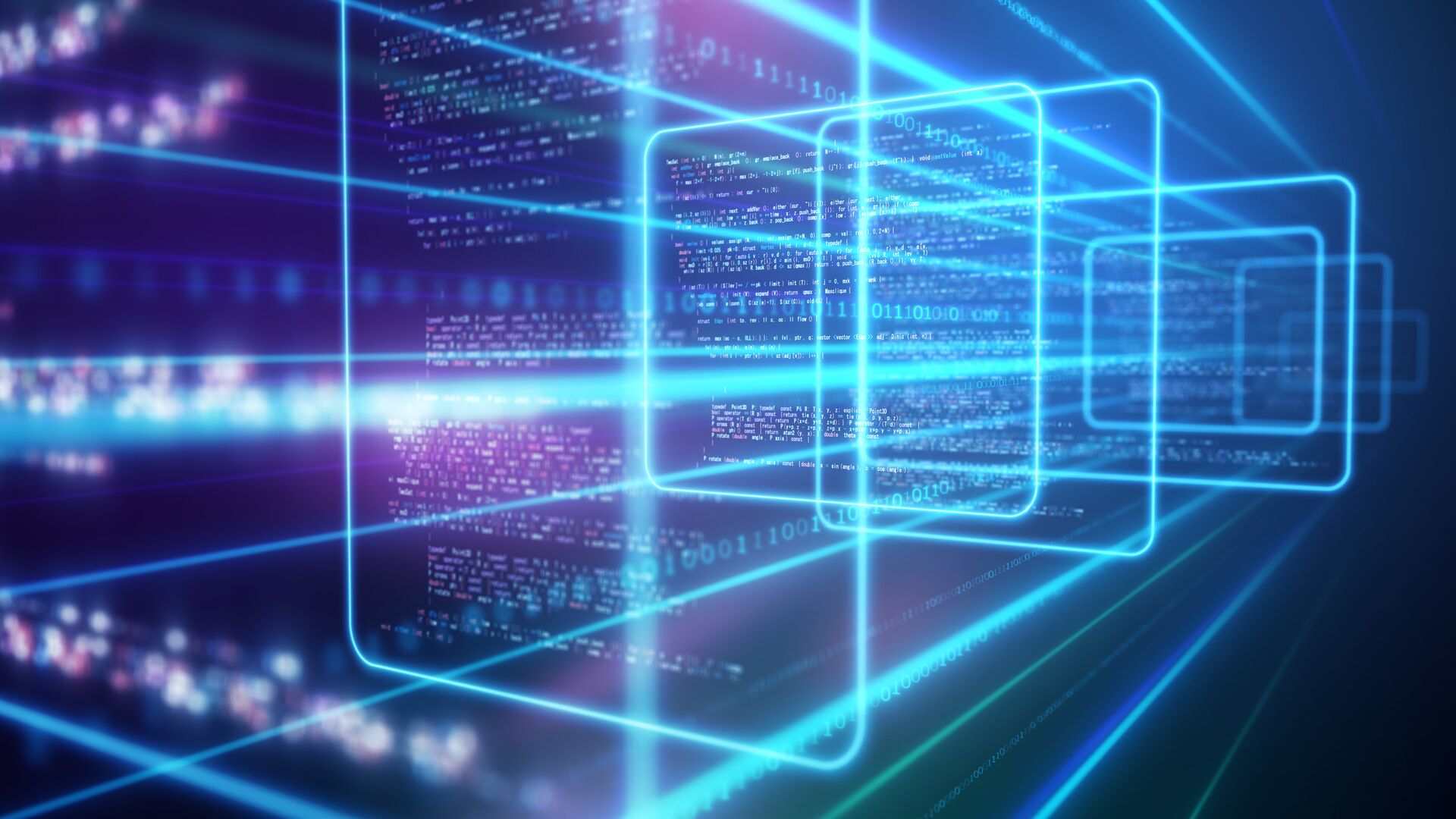
We view generative AI (GenAI) as a monumental tech shift on par with the internet, a once-in-a-generation tech shift, with broad implications for stocks and society. Our inaugural report on GenAI features proprietary analysis on productivity gains and TAM, market segmentation, and business models.
We also share insights into GenAI chatbots that utilize a Generative Pre-Trained Transformer (GPT) information source. In addition, we look at computer resources, hardware, data centers, cybersecurity, and the regulatory landscape.
GenAI carries the promise to revolutionize the ways humans and computers work together. While these breakthroughs have much more potential to replace worker functions than with past AI, we expect adoption to take place in the form of human augmentation and copilots, where computers work with humans. This will lead to a major step-function in productivity gains and another new architectural computing cycle for software.
Even more exciting is the fact that the rate of innovation in GenAI is the fastest the tech landscape has ever seen. We view this movement as a clear positive for tech investors as another innovation wave is upon us.
Our initial framework for potential productivity gains suggests $832B to $1.7T of US labor productivity gains. This will result in 8-16% of total annual US labor costs as the technology scales across most US industries in the coming years. We estimate companies creating GenAI technology could capture 20-30% of these savings, resulting in a GenAI tech spending TAM increase of $166B to $500B.
The current computational infrastructure fails to support largescale, large-language model (LLM) inference. To demonstrate these shortcomings, we calculated the theoretical ability of a leading CPU to generate large language model tokens versus a CPU’s ability to generate inferences from common smaller recommendation algorithms.
Today’s leading CPUs can generate only ~1% of the large language model tokens per second versus modern accelerators. This leads to our view that LLMs will lead to a paradigm shift in inference platforms to require acceleration over past CPU-based inference deployments.
We believe a new software stack will emerge, comprised of the LLM layer (akin to IaaS), the ModelOps layer (akin to PaaS) and the GenAI Apps layer (akin to SaaS).
We are conservatively estimating a ~1.2-1.3x uplift from GenAI as vendors offer solutions whereby computers create content and complete tasks on-demand for workers, governed and embedded into integrated apps and workflows. This would be similar to how the cloud drove a ~2x up-lift to on-prem software spend as it enabled companies to outsource internal hardware and labor costs. we would assume some pressure in gross margins as the cost to run GenAI models are higher, but techniques are getting more efficient and thus impacts are likely to be modest. We model GenAI software spending grow from <$1B in 2022 to ~$81B in 2027, representing a 190% 5-year CAGR.