Artificial Intelligence & Real Application in Retail With Focal Systems
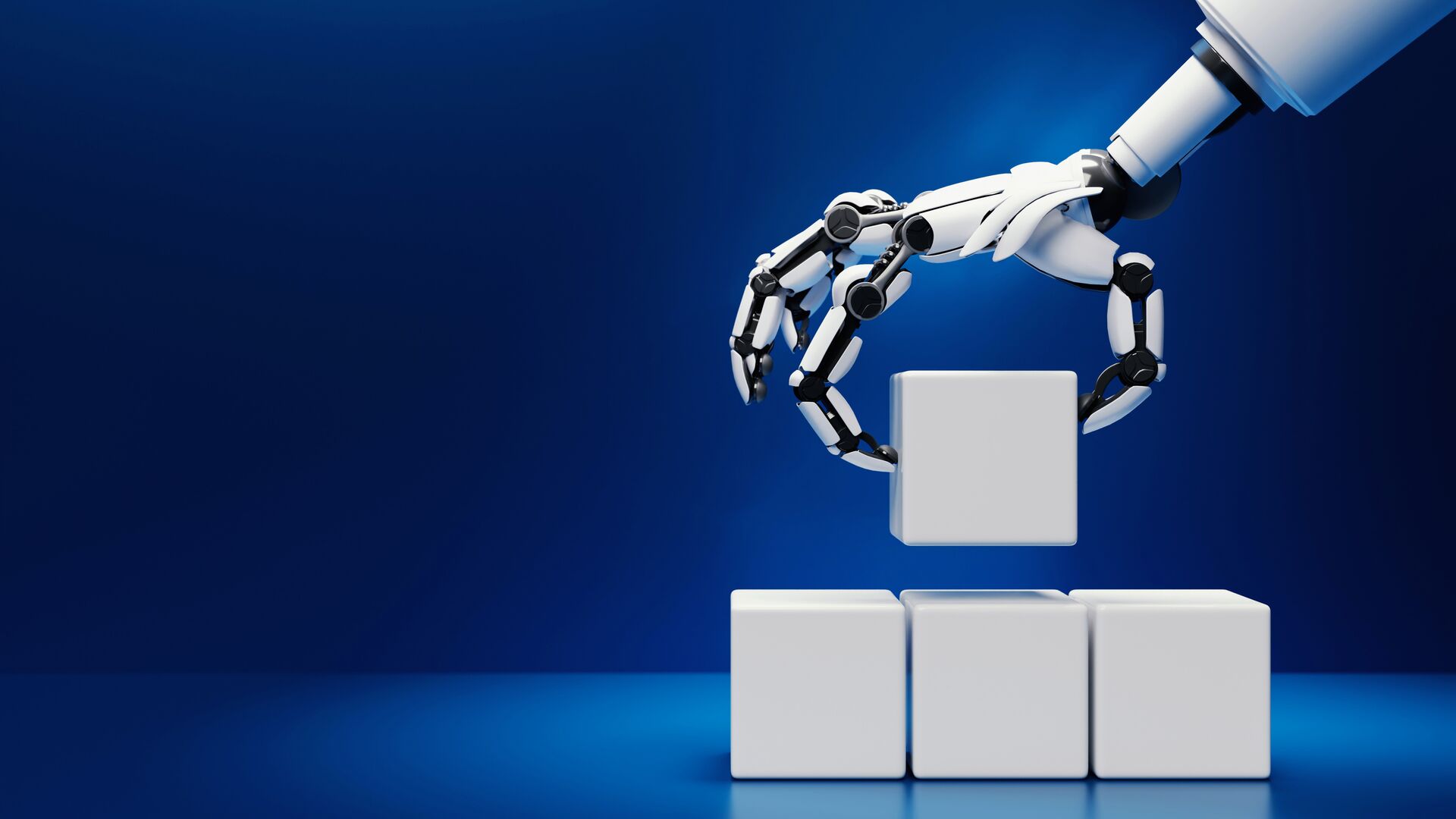
In this episode of TD Cowen’s Retail Visionaries Podcast Series, Francois Chaubard, Founder and CEO of Focal Systems, joins Oliver Chen, Cowen’s Retail & Luxury Analyst to discuss artificial intelligence applications in retail. They touch upon how artificial intelligence can optimize reordering, locate products in stores, reduce waste, and reinvent how retailers approach inventory planning.
Press play to listen to the podcast.
Narrator:
Welcome to Cowen Insights, a space that brings leading thinkers together to share insights and ideas shaping the world around us. Join us as we converse with the top minds who are influencing our global sectors.
Oliver Chen:
Artificial intelligence is changing the world in every single thing we do. Healthcare, automotive industry, visual search, and in a newer and earlier frontier retail, this is a visionary podcast series about visionary ideas and people. My name is Oliver Chen. I’m Cowen’s new platform retail and luxury analyst. We’re thrilled to have joining me for this episode. Francois Chaubard, the CEO of Focal Systems. It’s an important company with AI visual technology employed at Walmart and other major retailers.
I met him at Stanford. I’m a graduate of one of the most exciting AI programs there at the business school. Today we’ll dive into questions surrounding AI and retail. Use cases, what has and has not worked, and what’s the potential impact to P&L? Francois Chaubard is the founder and CEO of Focal Systems. He comes from an extensive background in deep learning and artificial intelligence after working at Apple’s Deep Learning Division, as well as creating and teaching two classes at Stanford. Focal Systems is a deep learning computer vision company focused on automating bricks and mortar retail through a realtime shelf digitization of the entire store. So Francois, thanks for being here with me. We’re really excited to have you.
Francois Chaubard:
Thank you for having me.
Oliver Chen:
How is artificial intelligence changing the world?
Francois Chaubard:
I think that it already has changed the world. If you look at advertising, you go online, you search for something that the ad that’s being shown to you, is an AI model, that Google ad sense ad words, baseball, the way that teams are selected. If you watch the movie Moneyball, speech-to-text service is a 9 billion industry, it’s like completely automated, translation services, agriculture, insurance, air travel. We have planes that can now land themselves. The Cirrus Vision Jet is the first publicly available. You can buy a plane that can land itself. I mean, you hit a button and it just does it. Self-driving cars, obviously that’s the biggest hype piece that Tesla now has beta nine, which is full self-driving capabilities. I think art and game development, art, AI just won its first art competition, right? I mean, it has already happened and it is just going to proliferate even further and impact every single piece of what you do today.
Oliver Chen:
So Francois, take us into retail. Where are we today with retail and AI? What are the biggest use cases people should understand?
Francois Chaubard:
Yeah, I think that retail is definitely lagging behind in AI adoption. There’s a lot of reasons for that. One of them I mentioned earlier on the last call, which is talking about they don’t have accurate realtime data. A prerequisite for being able to apply AI is having accurate realtime data. If you look at finance, right, if I’m trying to build an algorithmic [inaudible 00:03:23] algorithm and I have late data or inaccurate data, it’s going to make a lot of bad trades. And so, same thing is true in self-driving car. If you don’t digitize the world around the car and know that there’s cars coming at me or going away from me, whatever, then I’m going to crash a lot. If I’m 40 seconds late or even a minute late, I’m going to crash a lot. So in retail, that’s the first problem that we really needed to solve.
And I was shocked to know that PI accuracies were so bad. Inventory management accuracies were so bad. And if you’re trying to order accurately Cheerios and you don’t know how many boxes you have in the store, you’re either going to over order or under order, hard stop. And so the first step, you have to get accurate realtime data. And then once you have that, then you can begin to play the game of retail.
And that’s what we’ve basically done, Focals. We have two separate teams. One of them is focused on store digitization where we’re trying to digitize the four walls, the sales floor, the back room, the end of aisle to know exactly what’s there, the produce rack, the fridges, know exactly what is where in the entire store. Once you know that, the next group we have is the Focal OS team, is from that data, now automate order writing.
So I’m ordering just in time, reducing back stock, reducing shrink, reducing food waste. There’s a huge application there. And increasing availability, automatically writing the schedule for when people- stockers should arrive, what they should be working on and which priority, what the planogram should be a bunch different applications, but ones it all predicates on, you have to know what is where in the store.
Oliver Chen:
And Francois, talk to us about computer vision. How does that play into what you do and why is now the right time? What’s happening with the convergence of technology to make AI more viable right now?
Francois Chaubard:
Yeah, I think there’s two major tidal waves that we’re riding. The first one is the smartphone market. The smartphone market brought the cost of a two megapixel camera from, let’s say a hundred dollars before the iPhone, to $1.20. If you go on Alibaba right now and try to buy a two megapixel camera module, it costs $1.20. That is insane. And so with that, it brings down the cost of deployed computer vision by leaps and bounds, by a hundred x.
The next wave is what we can do with those images. If AI algorithms pre AlexNet, which is the big step function in the deep learning community, the most important AI paper of all time, in my opinion, pre AlexNet our error rates on any benchmark you would put, the benchmark that computer vision community uses is called ImageNet, I mean, I think the ImageNet accuracies were something like 20% at one accuracy. If I only get to make one guess, I’d be correct 20% of the time out of a thousand classes, now they’re at 91% accuracies at one accuracies, which is just insane. And so it’s leaps and bounds. So now it went from not useful at all, one out of five times I’ll get the answer right. Now, 91 out of a hundred times to get the answer. So it is completely of, those are the two massive waves that are coming that are going to have massive implications for driving self-driving cars, planes landing themselves, and reordering Cheerios.
Oliver Chen:
Francois, how has accuracy increased? The power of neural networks has become really evident.
Francois Chaubard:
I guess, let me talk about the AlexNet paper a little bit. Before the AlexNet paper, we had these very shallow models that were really simple and we would all handcrafted ways to try to find a cat in an image. And that’s basically what we were trying to do, the computer vision community was trying to do. And what happened in 2012 in Toronto, University of Toronto with these three guys, Alex Krizhevsky, Ilya Sutskever, and Geoffrey Hinton, is take GPUs, which were predominantly used for rendering triangles and video games, that’s like the main use case for GPUs in 2012. But it’s great at doing really parallelizable tasks like processing an image. I can start at every single pixel and run a really complex thing and loop over all pixels and do them at the same exact time in parallel. That’s why GPUs are so great.
And so now Alex Krizhevsky was a game developer. He applied Jeffrey Hinton’s idea of what’s called back propagation and neural networks, and back propagation is the way you train neural networks, and applied it onto a GPU, the first one to do that. And that showed the entire AI community a new way to get insane accuracies. And now the only limiting steps, there’s two. One is the size of the model. So do you have a big enough GPU that can handle the bigger and bigger and bigger and bigger models? That’s literally what’s blocking us from getting to even bigger, better AI. And two is access to realtime accurate data. And that’s why scale [inaudible 00:08:40] AI is worth a couple billion dollars in valuation, is like how do we get more accurate data? And once you get both those things, the floodgates open, it allows so many different things that you can possibly go and do. And so that’s really the two big step functions there.
Oliver Chen:
Francois, what about the P&L and retail, putting on your CFA hat, we both have CFAs, so what may happen with implementation of AI and the mechanics of the future of labor as well?
Francois Chaubard:
Yeah, so I think that there’s this lump labor fallacy that they teach in the CFA, which is like, Hey if there is X amount of labor inside in the world to make the economy tick and robots are doing more and more percentage up to a hundred percent, then what are we going to do? We’re all going to, I think, party, do art and there’s nothing for us to do. That just hasn’t been true. And I think that as you automate more stuff in retail, it doesn’t actually necessarily automate, reduce the amount of jobs that we’re going to ask people to do, now we can afford more people to go in and provide better customer service and things like that. So I think that now that’s one major trend. I think what actually happens, it makes the productivity of each stocker 10x more. And so that allows you to get maybe 10x more sales and get so much more.
And so it’s going to really, you’ll see kind of in dollar value, I think labor stays equal. I think in percentage of sales it goes through the floor. It goes from 13% sales to labor to maybe 5-6. And then what’s going to offset that, there’s going to be a little bit of offsetting with it, you’re going to have to pay for AI, which may be 1-3%, let’s say, depending on how aggressive you want to get in AI, of sales will go to AI. And then so that’s going to be, let’s say it’s five plus three is going to be 8%. You’re going to have an extra 5% of EBITDA margin. So you’re going to go from 3% EBITDA margins, which is typical in grocery, retail and non-inflationary environments to eight, which is a big, big, big deal.
Oliver Chen:
And Francois, what about the future of the store? The center of the store, curbside pickup, no checkout, automated checkout, just walkout. What do you see happening with these different variables?
Francois Chaubard:
Yeah, let me go in reverse order there. I guess the cashierless checkout, the Amazon Go flavor of that, I don’t believe in. I think that that is cost preventative right now. We worked on this when we were first starting out and basically you want to pick what your selectively permeable layer is. Is it at the till? Is it in the whole store? If it’s a whole store, it’s more expensive because you have to put these cameras everywhere. You have to track Oliver throughout the entire store and you have to use really expensive DSLRs and really real time expensive GPUs to process all that data. I don’t believe in that. I think that Amazon is kind of floundering right now with that use case. They tried, they said they were going to be in 3000 stories by 2021. Well it’s 2022, about to be 2023 and they’re in 54 is the last count I had.
So nowhere near 3000 and they cite the reason why is because of the cost of the system, it’s too expensive. GPUs are expensive and this is why Invidia stock is up so high. So I don’t believe in that one. I do believe in, just now taking AI out of the equation, just thinking about from a P&L perspective, if I have three different models, I can be, let’s call it a traditional grocery store that does not offer click and pick does not offer any e-com. I don’t let Instacart in my store. I don’t let DoorDash in my store. Model two is I’m a dark store, you’re not allowed to walk into my store. You can only order online and then it’s fulfillment, 15, 20 gorillas, all those guys. And then the third is a light store. And so I have a dark store and I open it up, Oliver’s allowed to walk in and buy stuff.
In this one, we already know the P&L, we’ve been doing it for a hundred years. We know what it looks like. In this one, it’s basically the same deal as Amazon. And we already kind of already know that P&L as well. I can’t walk into an Amazon fulfillment center and start picking stuff off the shelf. Now if I did, and let’s say I had a bunch of these all scattered and maybe they’re smaller, I don’t know, and now I just let Oliver walk in, that is at revenue accretive. Like someone was going to go to a grocery store, the first model and now, and they weren’t going to do the second one. And now in the light store, you will satisfy both customers, both use cases. Why would you not? And you have to pay the same rent. It’s the same rent either way.
So the highest return on assets, in my opinion, unquestionably is the light store model. Now how do you offer in a light store environment omnichannel? It’s very difficult. Do I have a separate section inside that store that is specifically for omni fulfillment centers? If you go into a Walmart, they’re doing that. They have little MFC sections in the store. Now I have five different places where Coke is, versus two, right? It just adds all this complexity. I can’t answer that question, but I do know that the economics, if I’m a grocer and I already have 5,000 grocery stores in the United States or something like that, like Walmart does, I’d make them all light stores. I would do fulfillment out of them.
And problem with this, and that we help solve, is they don’t know what’s in the store. So you go on to walmart.com, let’s say you order a hundred products, 25% are substituted out because they didn’t know that they didn’t have it in the store. That’s a terrible customer experience. The analog equivalent of that is you buy a hundred things in your basket, you’re pushing it to your car, cashier runs out after you, takes 25 products out, swaps them with something else that maybe you didn’t want. Are you kidding me? I mean, you’d never go back to Walmart again, but this happens.
Oliver Chen:
Yeah, there’s a lot of friction. You really want that apple, that special apple that you really want and asked for. I see what you’re saying with accuracy. But, so tell us a little bit about Walmart. What are you doing in the store now? What’s happening and why did Focal win versus other solutions?
Francois Chaubard:
We can only say so much. So I’ll try to say things that one could infer from public information. So you can walk into any store. Right now it’s chain wide. We’re doing chain wide rollout for all of Walmart Canada, which is 403 stores. It’s very exciting. We’re really, really, really excited to work with them. They’re thinking about it the right way. They’re changing the way that they’re talking about availability, using it from a focal OSSA versus their traditional systems and things like that. They’re thinking in terms of those OODA loops. We talked earlier, observe oriented, decide, act, and building those OODA loops based on the focal data, changing the way they order, changing the way that they are stocking, changing the way that they may be setting planograms or whatever, using all that data for all of that.
And it’s really exciting to be a part of that and help them and be their partner in digitization of the store. And then automation, which is a big piece of it. I think, there are a lot of retailers that are trying to build it themselves and we’ve seen, we talked to some of them and they basically had me on the whiteboard and it was like, explain what you guys do and I was like, okay, come on. You’re not buying. You’re trying to build. And we haven’t gotten that really at all from Walmart, which is great. And it’s been a great partnership. I’m really excited about that.
Oliver Chen:
Taking us back in our class at Stanford, and I’m also in the MIT program, supervised learning, unsupervised learning, reinforcement learning. Could you give us a quick take on what are those major buckets in terms of how they fit into the AI world and how might consumer retail use them?
Francois Chaubard:
Let me first add some color to what those terms mean. If you go to CS 229, they have great lecture notes on all of this, that will do way better than I will right now. Supervised learning is basically anytime I have a teacher or a supervisor telling the AI what to go and do. For us, we use supervised learning all the time, predominantly supervised learning, which is usually a labeler, is labeling an image saying, yes, that’s an out of stock. Okay, I train the AI. AI says that’s not out of stock. Oops, error, going back through the model, get better next time. Now you do get the answer right. Unsupervised learning is more anomaly detection [inaudible 00:17:01] a bunch of clusters and this little data point is not near any other data point. It’s probably anomalous, maybe it’s a spam, maybe it’s something else.
Reinforcement learning is mostly for gameplay. If you can whittle it down that the problem to a game. Chess, Go, checkers, I don’t know, even playing Dota, World of Warcraft, there’s a bunch of reinforcement learning in there and it’s trying to map a state that you could be in to an action and which action, if you have many actions, which action should it go take? And there’s advancements happening in all three fields for sure with deep learning, which is just bigger and bigger models. For applying those three to use cases of those three to retail, supervised learning, detecting out of stock from an image for sure. That’s a great supervised learning application. Unsupervised learning is fraud. If you detect that there’s some anomalous behavior happening and then maybe that’s someone trying to game the system and trying to do a refund, all of a sudden we see this giant amount of refunds in one store, right?
It’s like, why are we refunding $3 billion in one store? Kind of finding that anomalous behavior. This isn’t within the distribution we’d expect. And then reinforcement learning, I think this is the most exciting one in my opinion. If you have a Gameboy, you can go up, down, left, right, a, b. Those are your controls of the Gameboy and trying to win a game, Mario, whatever. How do I get retail down to a Gameboy? Where I control, what are my controls? I control the labor and the labor schedule. I control the SKUs that are in my store, what percent of the shelf they hold, shelf allocation or planogram. I control the forecast, how much I’m ordering and that’s about it. Advertising spends some other, that’s it. There’s not many variables that are in my control, that are control variables. So now you can give it, here’s the control. Go maximize EBITDA. Go maximize it.
Oliver Chen:
The other part of this is this magic plus logic IN product innovation and rethinking development. So taking it even a step back, I think, and the real side of creating a product you didn’t know you wanted or needed.
Francois Chaubard:
If I were CEO of a national retailer, this is what I would do. I would go down to a store, and I’d write down every single decision that a human is making and I would question whether or not we can make an AI to make it faster, better, more frequently and to optimize the full picture and maximize EBITDA quicker. And I would go and do that right now and then try to play the game.
Oliver Chen:
More broadly, it’s a great topic, what is your advice for CEOs? What are most C-suite coming to you? What are the biggest problems?
Francois Chaubard:
The knowledge base that a stock trader in 1970 needed versus in 2022 could not be more different. The overlap is maybe 3%, right? I mean it’s a completely different world. I think the same divergence is happening in retail. So I would advise very strongly that you go do the course that you did at Stanford where we met, go do that. Get smart on AI. You don’t need to know how to train your own models. I’m not saying that. I am saying that you should understand it, know how to deploy it, know how to use it. That’s what Jeff Bezos is doing for sure.
And if that’s your competition, it’s going to help all retailers no matter what. If you’re two stores in LA, if you’re a hundred stores in Vietnam, it’s going to help you make better decisions and increase EBITDA unquestionably. So you better get smart on it. And you can’t delegate that. They often, they hire a CAIO, right? Or a chief data officer. And so your job is to go figure out AI. No, you can’t do that. The CEO, it’s so important. It’s not a technology, it’s a strategy. And the CEO needs to be championing that from the top down.
Oliver Chen:
Francois, what about privacy concerns slash opportunities? What’s on your mind for that?
Francois Chaubard:
Yeah, I think this is a big one. That’s why we don’t get into, there’s a whole bunch of use cases for LP, loss prevention that I just do not, I’m not interested in because I don’t like the way that sounds. We’re going to be tracking people, identifying Oliver, and when Oliver, if he’s on my target list, I’m going to sound the red alarms and I’m going to do facial recognition in real time and send the alarms to the security guard, like go grab him.
Yeah, I’m kind of scared of that. I also think that Amazon has been struggling with that, with their Amazon Go platform. I mean now you have a hundred cameras that are very high resolution that are right in your face. I mean, you look up and you see this army of cameras that are right at your face. It’s very scary. We don’t get into that at all. We have the first model, and you can see this on the website, the first model, before we even save the image, cuts the person’s silhouette out of the image. We save the rest of the pixels because they’re useful. You can still detect a lot of out of stocks in them typically, but we do not want to worry about PII, personally identifiable information, or anything of the sort.
Oliver Chen:
And what do you think the nearest challenges will be in terms of what’s ahead with the new frontier? You know, what, what’s limiting growth slash pushing entities forward?
Francois Chaubard:
For retailers?
Oliver Chen:
Yeah, and the AI field at large. There’s been so much progress, but there’s so much opportunity ahead.
Francois Chaubard:
I would say the limits on the AI community are the size of GPUs and the availability of real time data. That’s the only limiting steps on those parts. Algorithmic complexity and things like that, we’re coming up with these new models that are just so cool and so powerful. But if you scale arbitrarily the GPU size and the amount of data that you have, the results just get better. Yeah, it’s like, it’s really insane the way it works. The limiting step from the retailer standpoint is an education gap. I think that the playbook, the people that are in charge and I say this with a huge amount of respect, know the playbook of the 80s, 90s, 2000s, which if you look at the Kroger-Albertson’s merger, right? Big deal, that’s the playbook. Growth by acquisition. If you’re trying to increase intrinsic value of the organization, you grow sales, you reduce costs, right? Figure out how to do that.
The ideas for reduction in cost is cut the labor, see what happens. I mean, that’s the strategy, is literally cut the labor, keep continuously… They’re not replacing it with technology. They really, sometimes, maybe it’s very… cash counting machine. I saw the most recent one was an automatic cake decorator. I mean, what are we talking about that’s like 0.01% of the labor or something like that? It just, it doesn’t flow into some AI strategy. And so I say again that the biggest limiting step in retail adoption is not knowing the new playbooks and not understanding AI enough to be able to devise an AI strategy. If you ask a CEO of a major retailer…
Oliver Chen:
I kind of want that cake thing. It sounds good. I want that. Last question, Francois, what about Web3 Metaverse and tokenization? Blockchain’s a big deal to me because it enables transparency. And as we study Gen Z and generation alpha, I think decentralization will be a key theme as well.
Francois Chaubard:
Yes. So, flashback to 1999, every company has studied with an e dash was going public at ridiculous valuations. And Allen Greenspan called this irrational exuberance, I think, is what it was called. It doesn’t mean you throw the baby out with the bathwater. And did we need ladders.com? Did I need a separate website to sell me ladders? No. But there were a couple companies that were going to survive the .com bust and became insanely the most important companies in the world right now, Google, Amazon, et cetera. Similarly, I think that 80-90% of this hoopla, I think goes away and burns off. It’s definitely in the recession coming in the next 12 months. But similar fashion, some of the most important companies are going to be formed in that space. Metaverse, I don’t really know, I don’t have too much of an opinion. I hope that we don’t live in a world where everyone just sits in their bed with a TV three inches from their eyeballs and never gets up and just has Amazon fly a drone to feed you.
And that’s kind of a dystopia in my mind, and largely I think that will lead to more isolation and more depression, in my opinion. I think that it’s a little bit scary. There’s some probably good, really good use cases for it that I like. Digital twins I think is a really big one like that I’m excited about. For Web3, decentralization, very exciting technology. We’ll see what the optimal use cases are for it that will survive. It won’t be all, I don’t really think the NFT thing. I have had my friends try to explain to me why Ticketmaster needs to be an NFT. I’m like, I don’t really get it. But relation to database can serve that function quite well. And it seems to be all right. Bitcoin, without question it, if every single view of, if you wrote down on a whiteboard, what are the things you want in a store of value?
In my opinion, I want it to be easily transportable. Okay, I have a zip drive and I can carry billions of dollars. I want to make it difficult to steal. I want to make it… A government can’t nationalize it. If we live in a great country, there’s a lot of countries that will nationalize people’s money, rich people’s money, and maybe take them into a prison and then steal all their money. If it’s in a zip drive versus in a bank, it’s a lot harder to steal. Ii it’s a land. Land is pretty easy to steal. So I think every measure, Bitcoin makes more sense as a store of value than anything else that we have out there.
Oliver Chen:
And Francois, final question. What AI is most exciting to you, are you most excited about? And any closing remarks you may have?
Francois Chaubard:
There’s two that I would really call out. The first one is called Stable Diffusion, is a new type of generative models that just won an AI competition. And if you look at these images, they are beautiful and they just won an art contest. First time an AI won an art contest. The judges didn’t know it was AI generated. It is just insanely beautiful, and the implication of this is that maybe AI can create movies, can create worlds that we can go live in. I mean, couple that with Metaverse, like why would I go deal with my, if I have a bad situation, I’m living in a trailer park, or why would I deal with that reality? I wouldn’t. I would just live in this crazy, really cool world.
The second one is large language models. There’s a couple of them. Palm, I think is talked about earlier, Pathway language model that Google produced. These models are insanely powerful and they can be applied to take the SAT and they can outperform. I don’t think that they’re going to get a 1600 on the SAT, but they’re like getting there. And it’s like it’s via just scaling up compute, scaling up the size of the models, and scaling up the amount of data fed into it. It’s really, really exciting.
Oliver Chen:
Yeah, stable diffusion can really unlock a lot of creativity and both of these technologies have that magic plus logic or we didn’t get into human in the loop, but enabling us to be different kinds of, hopefully better people and better businesses and more efficient businesses or big opportunities. Well, Francois, it was really fun to be here with you. Congratulations on the success of Focal Systems and thanks for providing us this deep learning experience and AI. We appreciate your time.
Francois Chaubard:
Thank you for having me.
Narrator:
Thanks for joining us. Stay tuned for the next episode of Cowen Insights.